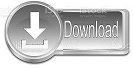
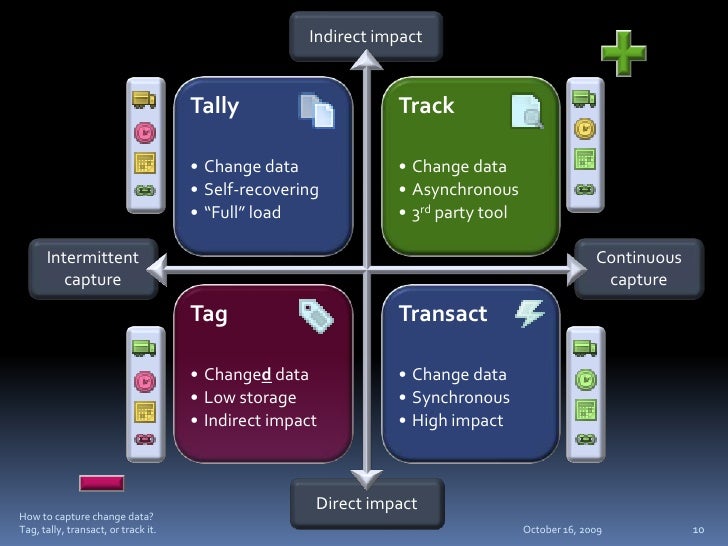
CDC is a very efficient way to move data across a wide area network, so it’s perfect for the cloud.Since CDC moves data in real-time, it facilitates zero-downtime database migrations and supports real-time analytics, fraud protection, and synchronizing data across geographically distributed systems.Log-based CDC is a highly efficient approach for limiting impact on the source extract when loading new data.
Change data capture windows#
Eliminates the need for bulk load updating and inconvenient batch windows by enabling incremental loading or real-time streaming of data changes into your target repository.Ultimately, CDC will help your organization obtain greater value from your data by allowing you to integrate and analyze data faster-and use fewer system resources in the process. Or even implementing a modern data fabric architecture. You may be moving data into a data warehouse or data lake, creating an operational data store or a replica of the source data in real-time. There are many use cases for CDC in your overall data integration strategy. This phase refers to the process of placing the data into the target system, where it can be analyzed by BI or analytics tools. ELT operates either on a micro-batch timescale, only loading the data modified since the last successful load, or CDC timescale which continually loads data as it changes at the source. In the more modern ELT pipeline (Extract, Load, Transform), data is loaded immediately and then transformed in the target system, typically a cloud-based data warehouse, data lake, or data lakehouse.

Today’s datasets are too large and timeliness is too important for this approach. Given the constraints of these warehouses, the entire data set must be transformed before loading, so transforming large data sets can be time intensive.
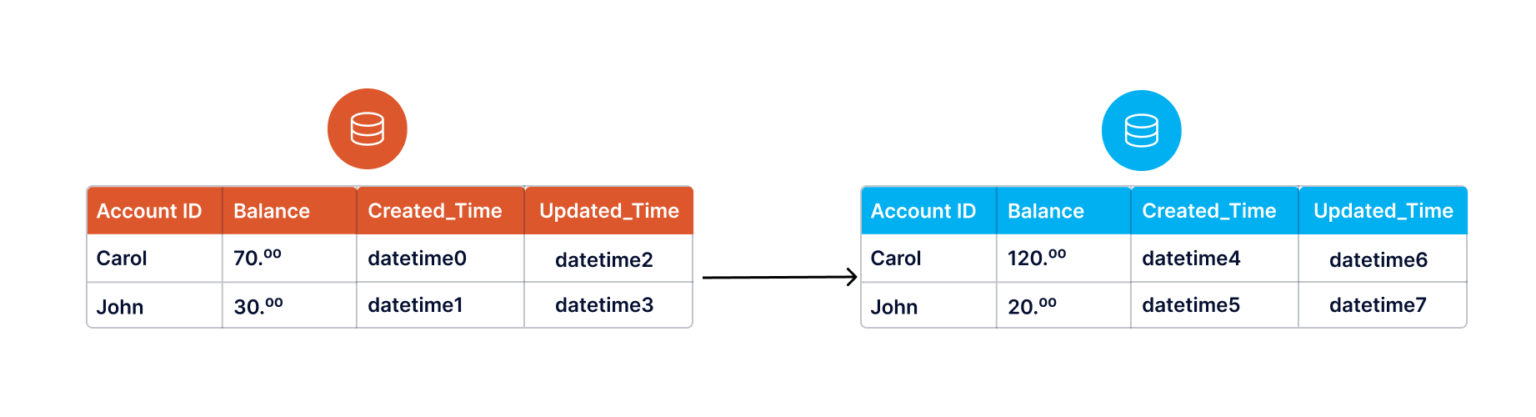
This involves converting a data set’s structure and format to match the target repository, typically a traditional data warehouse. Typically, ETL tools transform data in a staging area before loading. Completely refreshing a replica of the source data is not suitable and therefore these updates are not reliably reflected in the target repository.Ĭhange data capture solves for this challenge, extracting data in a real-time or near-real-time manner and providing you a reliable stream of change data. The challenge comes as data in the source tables is continuously updated. Historically, data would be extracted in bulk using batch-based database queries.
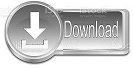